Data annotation: The key to effective KI
Discover how the Data annotation makes it possible to use AI and ML models more precise and effective to design.
What is data annotation?
Data annotation refers to the labelling of data sets with relevant information to make it easier for machines to understand. These data sets can consist of images, audio files, videos or text. By labelling data points in this way, machine learning (ML) models can learn effectively and recognise relevant patterns.
Why is data annotation important?
The continuous improvement of AI and ML models requires constant training. A decisive factor for success is correct data annotation, which ensures that models learn the right patterns and thus deliver precise results.
Advantages of data annotation
- Improve customer experience: Better interaction with chatbots and voice assistants through more human conversations.
- Increase safety and accessibility: IoT devices recognise noises and movements in the home, increasing security and accessibility.
- Accessibility for content: Online content such as videos and images are becoming more accessible for people with visual or hearing impairments.
- Improved speech recognition: Greater accessibility on mobile and desktop devices thanks to improved speech recognition.
Types of data annotation
- Image labelling: Objects in images are marked and classified using bounding boxes and segmentation masks.
- Text annotations: Text is tagged and metadata is added to recognise themes, moods and entities.
- Audio annotations: Transcription of speech with a focus on accents and demographic features.
- Video annotation: Tagging of video clips for object recognition and tracking.
Automated vs. manual annotation
- Automated annotation: Fast and inexpensive, but can be inaccurate.
- Manual annotation: More precise, but time-consuming and more expensive.
Challenges and best practices
- Challenges: Time-consuming and accurate labelling and ensuring that all data is correctly marked.
- Best Practices: Implementing efficient data entry pipelines, ensuring annotation tools meet requirements and training staff for effective annotation
Data annotation is a crucial process that enables ML models to learn more accurately. Organisations should invest in the right combination of automated and manual methods to obtain high-quality data for machine learning.
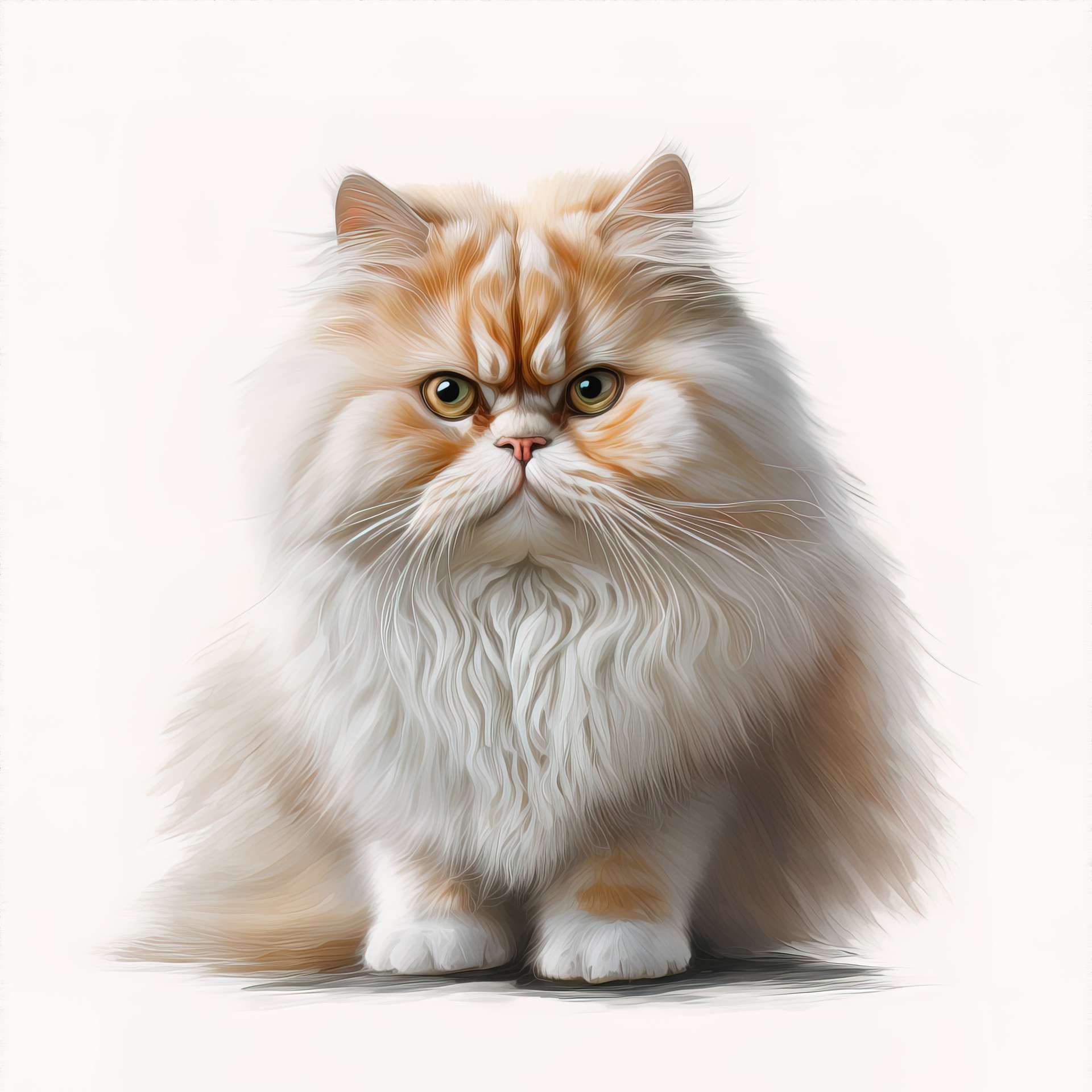
Entdecken Sie, wie die Datenannotation es ermöglicht, KI- und ML-Modelle präziser und effektiver zu gestalten.
Was ist Datenannotation?
Datenannotation bezieht sich auf das Kennzeichnen von Datensätzen mit relevanten Informationen, um Maschinen das Verständnis zu erleichtern. Diese Datensätze können aus Bildern, Audiodateien, Videos oder Text bestehen. Durch diese Markierung von Datenpunkten können maschinelle Lernmodelle (ML) effektiv lernen und relevante Muster erkennen.
Warum ist Datenannotation wichtig?
Die kontinuierliche Verbesserung von KI- und ML-Modellen erfordert ständiges Training. Ein entscheidender Faktor für den Erfolg ist die korrekte Datenannotation, die sicherstellt, dass Modelle die richtigen Muster lernen und damit präzise Ergebnisse liefern.
Vorteile der Datenannotation
- Kundenerlebnis verbessern: Bessere Interaktion mit Chatbots und Sprachassistenten durch menschlichere Konversationen.
- Sicherheit und Zugänglichkeit erhöhen: IoT-Geräte erkennen Geräusche und Bewegungen im Haus und steigern so Sicherheit und Barrierefreiheit.
- Barrierefreiheit für Inhalte: Online-Inhalte wie Videos und Bilder werden für Menschen mit Seh- oder Hörbehinderungen zugänglicher.
- Verbesserte Spracherkennung: Größere Zugänglichkeit auf Mobil- und Desktop-Geräten durch verbesserte Spracherkennung.
Arten der Datenannotation
- Bildbeschriftung: Mit Begrenzungsrahmen und Segmentierungsmasken werden Objekte in Bildern markiert und klassifiziert.
- Text-Anmerkungen: Texte werden mit Tags und Metadaten versehen, um Themen, Stimmungen und Entitäten zu erkennen.
- Audio-Kommentare: Transkription von Sprache mit Schwerpunkt auf Akzente und demografische Merkmale.
- Video-Annotation: Markierung von Videoclips zur Objekterkennung und -verfolgung.
Automatisierte vs. manuelle Annotation
- Automatisierte Annotation: Schnell und kostengünstig, kann jedoch ungenau sein.
- Manuelle Annotation: Präziser, aber zeitaufwändig und teurer.
Herausforderungen und Best Practices
- Herausforderungen: Zeitaufwändige und genaue Kennzeichnung sowie sicherstellen, dass alle Daten korrekt markiert sind.
- Best Practices: Einführung effizienter Dateneingabe-Pipelines, sicherstellen, dass Annotationswerkzeuge den Anforderungen entsprechen und die Ausbildung des Personals für effektive Annotation
Datenannotation ist ein entscheidender Prozess, der es ML-Modellen ermöglicht, genauer zu lernen. Unternehmen sollten in die richtige Kombination aus automatisierten und manuellen Methoden investieren, um qualitativ hochwertige Daten für maschinelles Lernen zu gewinnen.